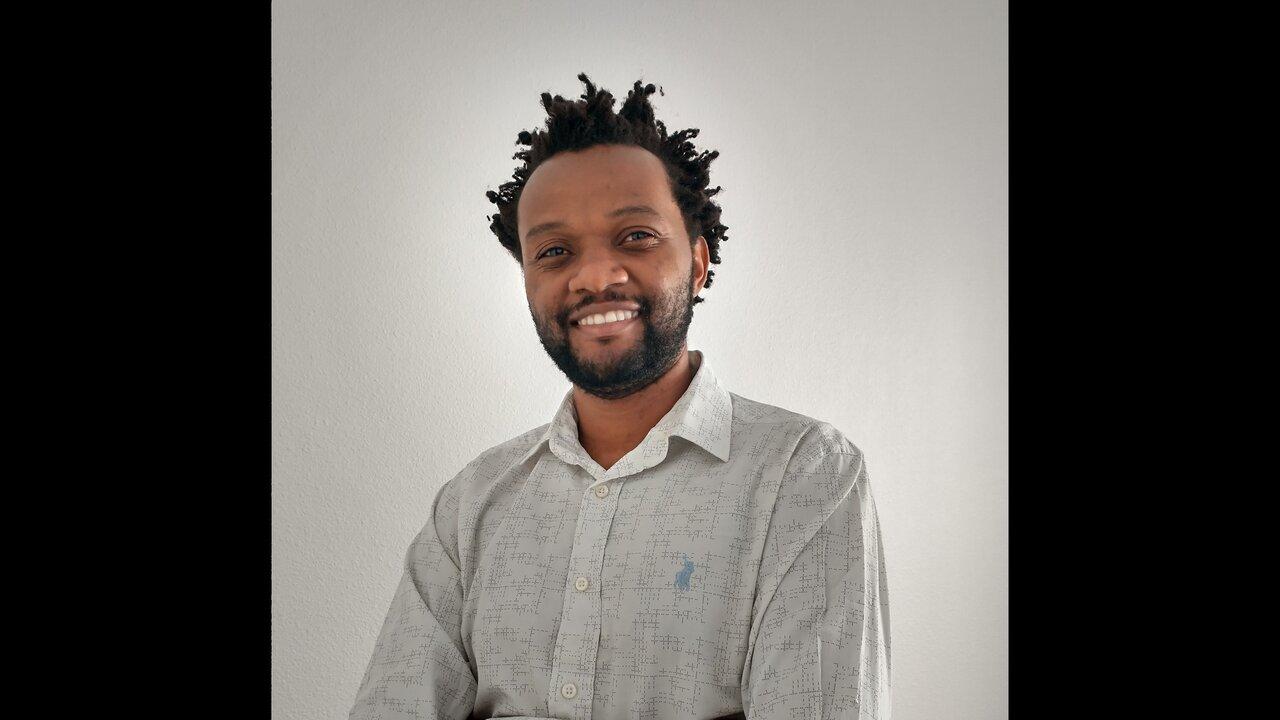
Simbarashe Nyatsanga Receives IBM Ph.D. Fellowship for Research in Gesture Generation
Quick Summary
- Nyatsanga is one of 16 recipients worldwide of the prestigious computer science Ph.D. fellowship
- Using randomly-generated gestures in animation and computer vision can improve interactions with characters, virtual agents and robots by making them feel more natural
Computer science Ph.D. student Simbarashe Nyatsanga aims to make an impact in animation and computer vision through his groundbreaking research in speech-driven gesture generation. He is one of only 16 recipients worldwide this year of the prestigious IBM Ph.D. Fellowship Award, which recognizes outstanding Ph.D. students with demonstrated academic excellence and expertise in pioneering research in computer science.
“In animation, there’s a lot of room for improvement, so it’s wonderful to see that IBM recognizes the importance of my research,” he said.
All humans gesture, whether it’s moving their arms while talking, or using their hands to say, “come here.” It is an essential part of communication, but translating these concepts to machines is challenging. With his IBM-supported research, Nyatsanga will tackle two different aspects—how to transfer gesturing style from one speaker to another in animation and how to use gestures to communicate with robots.
“I am interested in these two ways of using gestures,” he said. “One which is expressive and stylistic, and the other which is well-defined and has a specific semantic meaning that a companion has to follow.”
A New Layer of Expressive Animation
On the animation side, Nyatsanga will leverage publicly-available data on gestures of televangelists, lecturers and talk show hosts, both when they are standing and sitting. He plans to develop animation algorithms across this wide range of contexts and styles and see if he can apply the gesturing style of one speaker onto the speech of another—for example, John Oliver’s gestures onto a Barack Obama speech or vice versa.
“This would give artists the ability to experiment with some gesturing pattern before editing the animation to convey a particular emotion,” he explained. “They already do this with facial animation, where you can express fear, excitement, happiness or sadness. My research builds upon that with conversation gestures and adds another layer of emotion.”
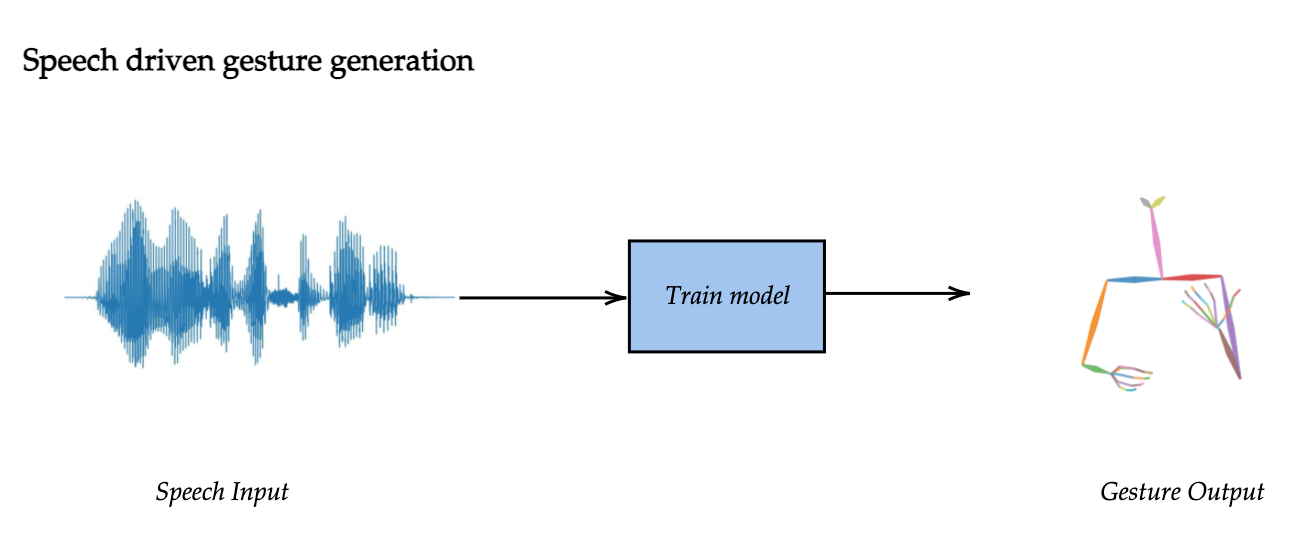
Nyatsanga sees the potential to incorporate these breakthroughs into industry-standard animation software like Blender and Maya. The ability to crowdsource gestures from YouTube videos could unlock new tools for animators to incorporate different styles into their characters. Moreover, improved gesture animation can enhance production in video games, animated movies and virtual assistants by conveying emotion and making them feel more natural.
“Humans can say the same sentence twice, but gesture differently both times, so there’s a great deal of natural and unpredictable variation that we experience day-to-day when we communicate with people,” he said. “My focus is understanding how to use stochastic or probabilistic models to encode this kind of variation in a way that would seem natural.”
One possible application is real-time character interaction with platforms like Siri, Alexa and Google Assistant that are currently disembodied. Stochastic algorithms can potentially be used to add animated or robotic embodiments to any of these systems in a way that’s not possible by hand animation due to the complexity of the systems. This could improve the interactions by making them feel more natural.
Communicating with Machines
The other part of the project is using gestures to communicate with robots. In other words, putting a hand up could tell a robot to stop, or motioning to come forward would instruct it to come forward. Control gestures represent a significant step forward in human-robot interaction, with applications in caregiving, self-driving cars, rescue missions in hazardous environments and space exploration.
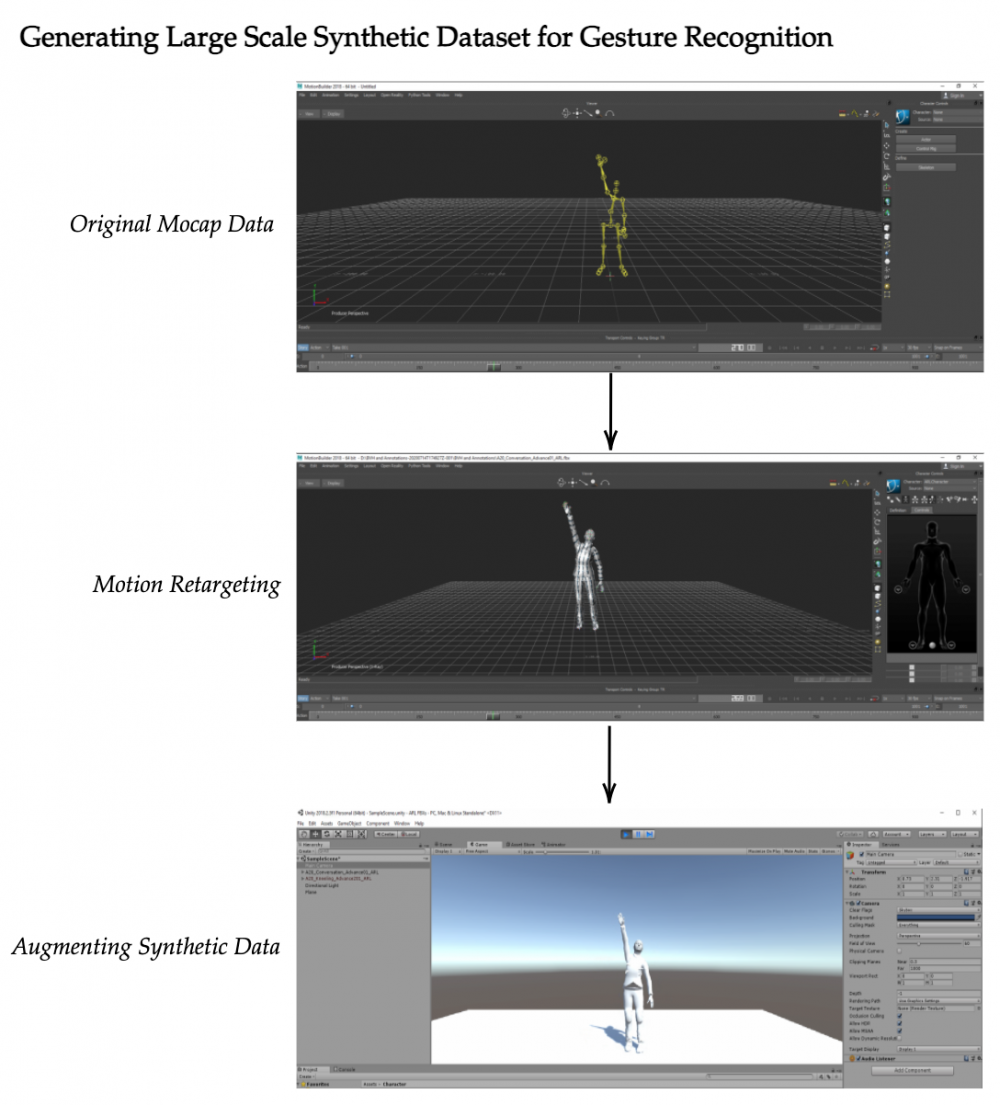
Teaching this to machines, however, involves the two-pronged challenge of getting the machine to recognize the gesture and then correctly interpret it. This requires collecting a lot of high-quality training data for each gesture, which itself is a manual, time-consuming and error-prone process.
To solve this challenge, Nyatsanga will collect motion capture data of participants performing a set of control gestures to establish a small “ground-truth” data set. Then, an algorithm will learn to imbue the natural motion characteristics of the ground-truth dataset onto a synthetic dataset that’s large enough to train machine learning algorithms.
Since coming to UC Davis, Nyatsanga has been grateful for the support of his advisor, Computer Science & Cinema and Digital Media professor Michael Neff, who he says has been a champion of his ideas. He plans to continue his research after finishing his Ph.D., focusing on developing generative algorithms that incorporate multiple factors such as speech, text and spatial context.
“What’s interesting about this problem is that there’s a linguistic link between spoken word and gesture that is not fully understood yet,” he said. “Realizing that link through character animation can help enrich storytelling and improve human-robot interaction through non-verbal communication.”