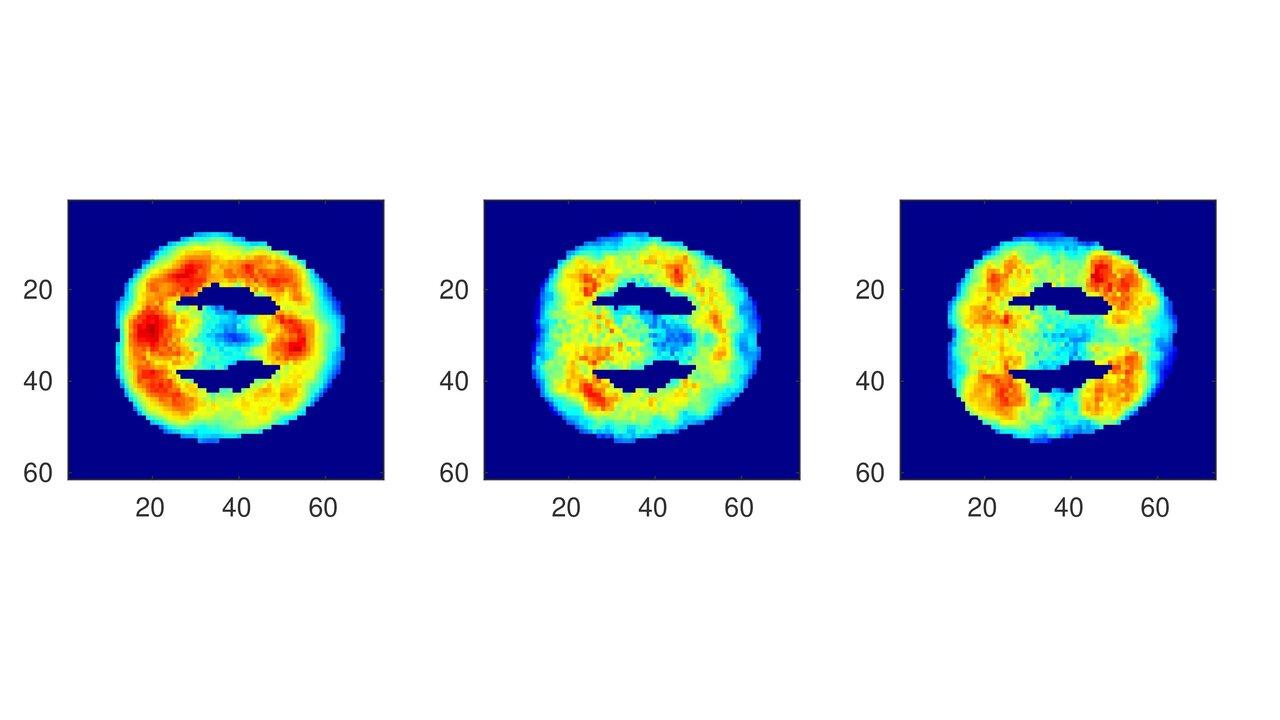
Using AI to Treat Teenagers With Schizophrenia
Computer science professor Ian Davidson will bring expertise in explainable artificial intelligence (XAI) and deep learning (DL) to a new National Institute of Health (NIH)-funded project to treat teenagers with schizophrenia. The five-year, $3.4 million project funds trials using AI to prescribe treatments for each patient based off medical images of their brains. The project is led by UC Davis School of Medicine professor Cam Carter with co-PIs Davidson and Tyler Lesh, John Ragland and Tara Niendam from the Department of Psychiatry and Behavioral Sciences.
Precision treatment
While schizophrenia is manageable with proper treatment, everyone’s brain operates differently, meaning some treatments will work for some people, but not for others. Currently, finding the right treatment requires long test periods to determine whether they are effective. This is especially a problem for young people with schizophrenia, as the sooner they are treated, the better the outcomes.
“These are big issues that are helping some of the most disadvantaged people,” said Davidson. “And given the right circumstances and data, we think a machine can potentially do a better job than a human.”
The clinical trial will enroll adolescent schizophrenia patients, who will have their brains imaged for the AI to use to recommend a treatment. The AI will be trained by being shown these brain images alongside the treatments that did or did not work for each patient.
“This idea of precision medicine relies on data to make decisions,” he said. “We are applying deep learning to learn what types of people’s brains respond to what types of treatments.”
The team hopes the machine can find patterns and be able to quickly recommend the most effective treatment to new patients. If effective, the technology will help doctors give patients the treatment they need faster.
Explainable AI (XAI)
As part of the project, Davidson’s team will tackle two challenging problems in AI. The first is making sure the machine can communicate why it made the treatment recommendation it did for each case. Doctors need to be able to validate the results and ensure fairness and patients need to understand the factors that influenced the decision for the sake of transparency.
The other challenge is what’s known as the small data problem. The AI in this study has to learn from only a couple hundred data instances, as opposed to the millions it would get if it were looking at something like data from Google. Their goal is to find ways to make up this gap, including teaching the AI to generate its own virtual patients that it can then train on.
“It’s a hard problem to deal with, but one way we’re looking into solving it is to teach the machine how to generate virtual instances so it can augment the real patient with the virtual patients,” he said.
Though Davidson’s group has done a lot of fundamental research in both topics that has been funded by Google, the National Science Foundation and the Office of Naval Research, this study is taking it to the next level.
“It’s a good example of basic research being applied to a real situation with real people,” he said.
The funding for the grant titled, “Pathophysiological Biomarkers of Treatment Response in Early Psychosis,” began July 1.